The QuCal Lab, housed in the Physics Department of Amirkabir University of Technology, focuses on advancing quantum technologies through interdisciplinary research. Our main areas of study are quantum computing, quantum machine learning, and quantum applications in chemistry. We aim to develop novel quantum algorithms and learning models, simulate quantum systems, and explore real-world applications in science and industry. By combining theory and implementation, the lab is committed to pushing the boundaries of quantum computation and contributing to the future of intelligent, quantum-enhanced technologies.
Quantum Computing
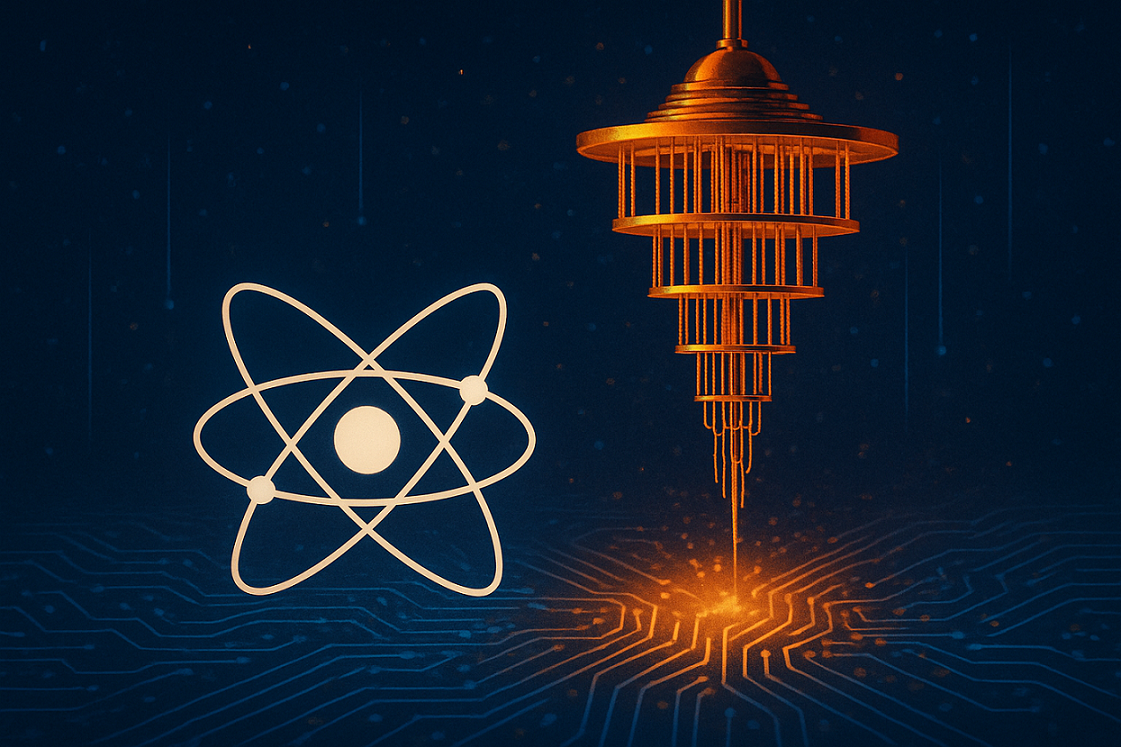
Our research in Quantum Computing centers on the theoretical and practical development of algorithms and architectures that harness the unique properties of quantum mechanics to perform computation. We investigate a wide range of topics, from the design of high-level quantum programming languages and the development of efficient quantum error mitigation techniques to the study of quantum circuit complexity and quantum information protocols. A major thrust of our work lies in understanding how different quantum models—such as gate-based quantum computing, Ising machines, and variational quantum algorithms—can be used to solve classically intractable problems. We are particularly interested in the scalability and fault-tolerance of quantum systems and the interplay between physical hardware constraints and algorithmic performance. This includes research into quantum simulation, quantum communication, and hybrid quantum-classical systems. Our lab regularly evaluates new frameworks and compilers, contributing to the growing ecosystem of quantum development tools. By bridging the gap between quantum theory and implementation, our work in this area aims to accelerate the realization of useful quantum computers and provide a robust foundation for their integration into scientific and industrial workflows.
Quantum Machine Learning
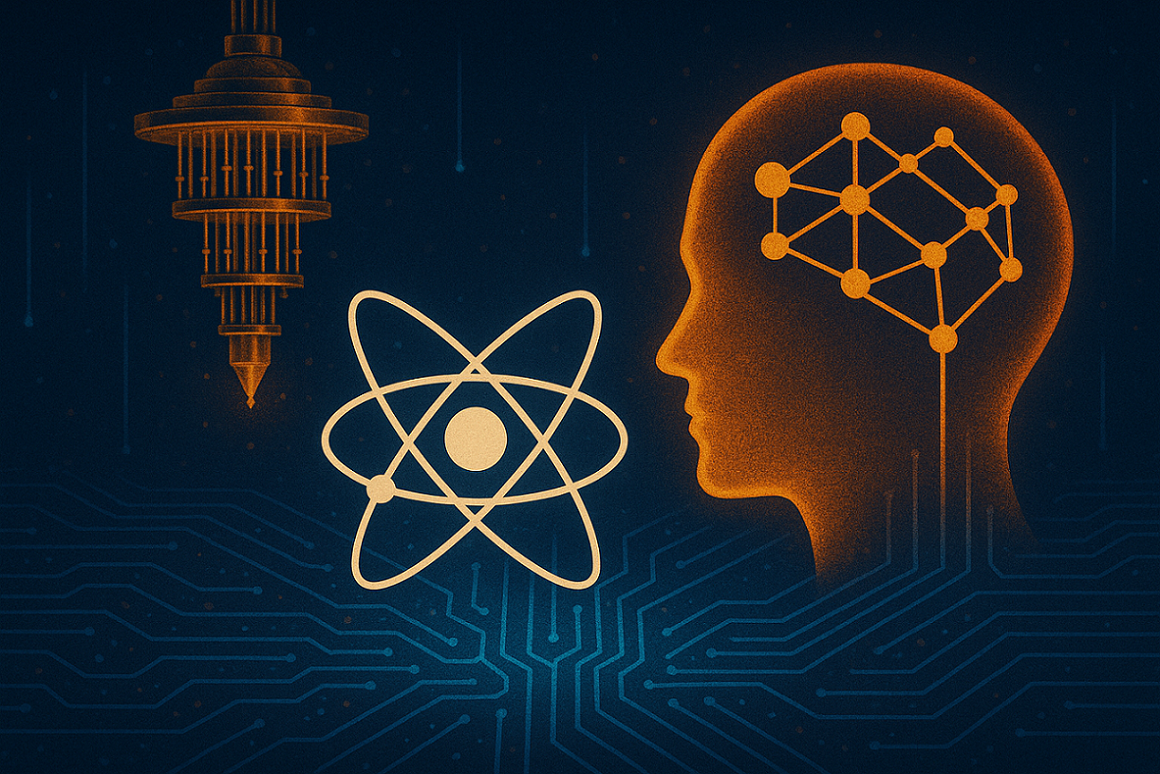
At QuCal Lab, our research in Quantum Machine Learning (QML) investigates how quantum systems can enhance and transform the way machines learn from data. We explore the use of quantum circuits to represent and optimize learning models, leveraging quantum entanglement and superposition to encode complex patterns that would be difficult or impossible for classical systems to capture efficiently. Our work includes the design and analysis of variational quantum algorithms, quantum kernels for classification, and hybrid quantum-classical models for both supervised and unsupervised learning tasks. One key area of focus is the use of quantum-inspired methods to train energy-based models such as Restricted Boltzmann Machines (RBMs), which are particularly well-suited for representing probabilistic data. We also study the implications of quantum learning theory, including the capacity and generalization bounds of quantum models. Through simulation and experimental implementation on near-term quantum devices, our team evaluates the performance and scalability of these techniques. The overarching goal is to identify and develop quantum algorithms that not only outperform classical methods but also offer new paradigms for data representation and inference, ultimately contributing to the long-term vision of quantum-accelerated artificial intelligence.
Quantum Chemistry
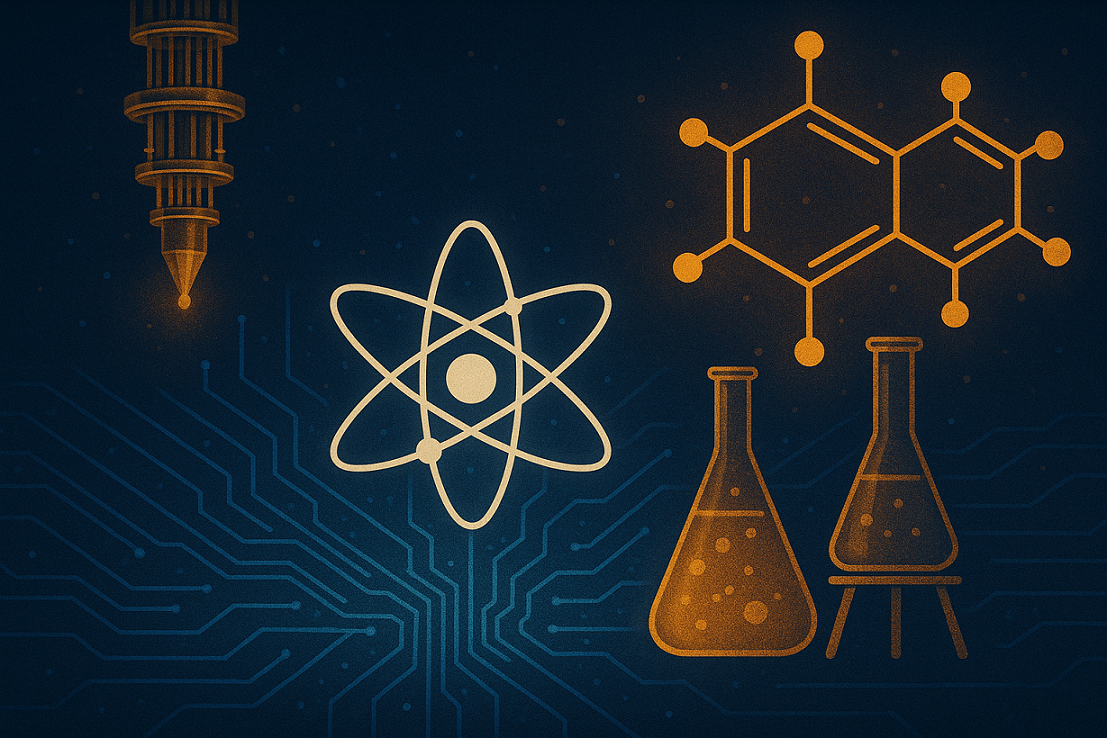
QuCal Lab also conducts cutting-edge research at the intersection of quantum computing and chemistry, aiming to revolutionize the way we simulate and understand molecular systems. Traditional computational chemistry techniques face scalability challenges when modeling the electronic structure of large and complex molecules. Quantum computing offers a promising alternative by exploiting quantum bits to natively represent the behavior of quantum systems. Our research focuses on developing and refining algorithms such as the Variational Quantum Eigensolver (VQE) and Quantum Phase Estimation (QPE), which are tailored to simulate ground and excited states of molecular Hamiltonians. We work on improving the accuracy, convergence, and noise resilience of these algorithms on near-term quantum devices, while also collaborating with chemists to apply them to real-world problems such as reaction dynamics, catalysis, and material discovery. Additionally, we explore quantum-inspired models that approximate chemical behavior using classical resources informed by quantum principles. By bridging the disciplines of quantum information science and theoretical chemistry, we strive to unlock new insights into the molecular world and pave the way for quantum-enabled discoveries in medicine, energy, and materials science.